How is AI being used in medicine and healthcare?
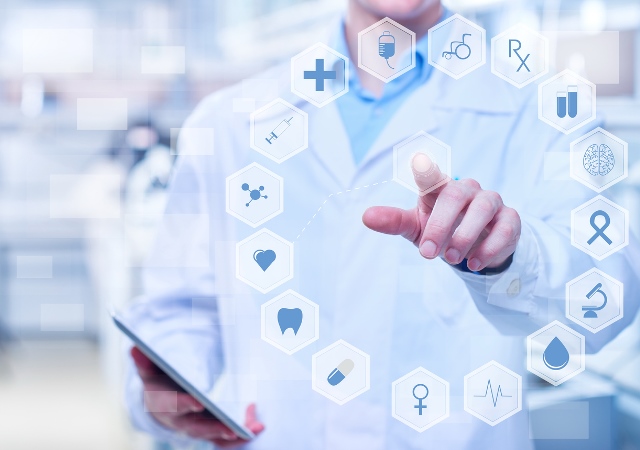
Virtually all active diagnostic devices that use software to interpret sensor data could be expected to benefit from incorporating AI into their controlling software. Devices that are deemed to be “good enough” might, of course, not need to be improved. But in principle, devices that can capture and make use of more information about a patient’s immediate physiological state, or emerging response to treatment, might be expected to yield better patient outcomes. It is in this setting that increased use of AI can be predicted. For an excellent example, consider the use of glucometers in controlling diabetes, and possibility of optimising insulin delivery (Atlas et al., 2012).
If we were to look at where AI is beginning to have commercial impact, we can look at systems that make intensive use of computation: imaging systems are a good example. Here, patient benefit might primarily lie in the use of assistance in the diagnostic process, and there are several examples. Radiomics is the most high-profile of these (O’Connor et al., 2017), where operators are applied to images to extract quantifiable measures to be used as biomarkers. Biomarkers computed in this way – known as imaging biomarkers – are being suggested to perform patient stratification in order that appropriate therapies can be given (Valdes et al., 2016). Cancer Research UK (CRUK) and the European Organisation for Research and Treatment of Cancer (EORTC) have recently produced a biomarker roadmap (O’Connor et al., 2017) to accelerate clinical translation of imaging biomarkers. One of the potential uses is in patient stratification (Parmar et al., 2015a; Parmar et al., 2015b), but other uses exist in early evaluation of treatment effects, and even determining surgical margins (Taylor et al., 2014); both are potentially critical to patient outcome, and there is increasing reliance on machine learning to implement image-based biomarker detection or measurement (Parmar et al., 2015a; Parmar et al., 2015b).
We can also identify situations where new measurement capabilities are enabled by AI. Free-breathing MRI (FB-MRI) scanning (Tison et al., 2018) is one of these, where there is clear patient benefit. In FB-MRI, optical flow1 algorithms are applied to correct for chest wall and cavity motion. Existing systems on the market are unlikely to employ algorithms for optical flow based on machine learning. But ANNs, such as FlowNet 2.0 (Ilg et al., 2017), exceed the performance of known hand-engineered algorithms for computing optical flow, and such networks are likely to replace human-designed algorithms in the future.
Looking at the other end of sensor complexity, we can consider rather less sophisticated data capture techniques, and the potential in collecting clinical and laboratory measurements and patient outcomes at a very large scale. Here, it is more likely that techniques of machine learning will also be employed: the quantity of data supports learning, and large-scale data acquisition allows both device level and patient level peculiarities to be “averaged out”. Inference of patient risk can be finessed in a way that has only – until recently – been achievable with epidemiological studies, or very large-scale clinical trials. Perhaps the best known deployment of this is the feature of Apple Watch 4 for the detection of atrial fibrillation (AF). A proof of concept of AF detection with sensors on Apple Watches, using data from more than around 9,000 patients, suggests that a deep neural network, specified by just over half a million parameters, was able to infer the presence of AF with moderate-to-high degrees of accuracy (Tison et al., 2018).
The US National Academies of Sciences, Engineering and Medicine have recommended several steps toward improvements in diagnosis: emphasis on early and correct diagnosis of conditions, reduction in diagnostic errors, and the avoidance of “overdiagnosis” (Balogh, Miller & Ball, 2015). Encoding the diagnostic decision process has traditionally been done by carefully designed diagnostic decision trees, informed by clinical expertise, and experience. Examples of such systems are now widely available, open to the public, in the form of online “symptom checkers” (Semigran et al., 2015; Semigran et al., 2016). These include those that are based solely on patients’ observations, and some that even support inclusion of blood test results. Most of these systems are static, and non-probabilistic: they do not learn from outcomes. They are known to have deficiencies, some of which can be corrected by adopting machine learning approaches, natural language processing and maintaining histories on the tendencies of individual patients.
This is an excerpt from the white paper Recent advancements in AI - implications for medical device technology and certification. To download our other medical device white papers, please visit the Insight page on the Compliance Navigator website.
Request more information today for a call back from a member of our sales team so that you can get a better understanding of how Compliance Navigator can meet your needs.
The Compliance Navigator blog is issued for information only. It does not constitute an official or agreed position of BSI Standards Ltd or of the BSI Notified Body. The views expressed are entirely those of the authors.